Studying Architecture After AI
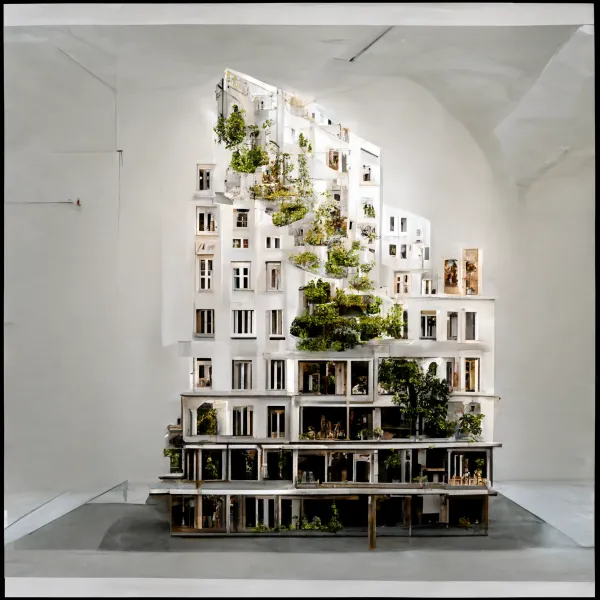
Why should one study architecture if anyone can design anything anywhere instantly at any time? Today—much faster than predicted—anyone with access to a cellphone can draw anything of their desire at a resolution indifferent to reality. The implications for architecture are obviously ground-shaking. This essay tries to grasp some of it—its implications for teaching and learning in a studio environment, and for preparing students for the significant challenges that face architectural practice today.
For hundreds of years, and in Western cultures since the publication of Alberti’s treatise De re aedificatoria in the mid-fifteenth century, architectural knowledge was defined as a notational science. Architects invented ways to notate, draw, compose, or codify—not to build (that was the role of the craftsperson) nor to house (that was the realm of the client). The role of the architect was only to mediate between both. Plans, sections, and other architectural representations quickly notated comprehensible forms on one hand, and economic—and, later, cultural—investments on the other: the matter and methods to build. Made by humans for humans, buildings had to be easy to understand. If a client's intentions were easy to understand, more complex shapes could be built with less. A symmetrical drawing was considered beautiful because the mirrored half of an original was only half as difficult to understand, which, above all, halved the cost of planning and making.
Deeply linked to the economics of a building, the composition of a building, therefore, served as a kind of benchmark to value the design. Aesthetics equated with the utility of a building. Alberti identified three aspects that shaped research in architecture for generations to come: first, a beautiful design uses first a limited number of parts which keeps the needed equipment and workers on a construction site manageable; second, those parts should have a clear form that is easy to produce and transport; and finally, those parts should be composed into a correlated arrangement using symmetries and other connotations to make it easier to comprehend the building. Since then, research in architecture invented notation after notation to design more with ever less. The Baroque celebrated architects who could draw complex forms by calculating the transformation of simple shapes. In very similar ways, five hundred years later, the Digital celebrated architects who could shape any free form from even less than parts: particles.
Alberti’s treatise did not contain any drawings, images, or other notations. For Alberti, ultimately, an architect, like a god, would form matter with words. And here we are today: Networks like OpenAI’s DALL·E 2 draw realistic images from text descriptions only. A digital image is built from pixels, two-dimensionally arrayed square-y points. Technically, it takes very little additional information to turn a pixel into a voxel and an image into architecture. In limitlessly replicable ways, the network can be applied to anything at any place instantly, turning anyone with the desire into Alberti’s ultimate architect and Harari's Homo Deus. By that, architecture and a research project that spanned over five hundred years comes to an end. When natural language can process three-dimensionally, there won’t be any need for an extra architectural language; for any plans, sections, or similar mediations that translate intention into form.
Natural language models translate not only the desires of clients but anything that is easier to communicate through words—like communal decisions, legislative codes, and, in general, any kind of knowledge. The superiority of AI models is already beginning to shatter. For example, the text-based model GPT4, which is readily available through a number of free online writing tools, utilizes over one hundred trillion different parameters. By comparison, the average adult uses only up to thirty thousand different words. That means a neural network uses three billion unique assets per one word you use. By looking at vast amounts of data, digital machines learn to repeat correlations between different features, which then can be interpolated and automated. Thus, AI can automate any task that is particular enough to be identified as such and that is frequently executed.
Despite Richard Sennet’s famous expression that “for great craftship, it needs first a passion and then 10,000 hours more of it,” any profession that relies on classic crafts will most probably be automated. Subsequently, architects will have to find new ways to contribute as a profession by valuing a different set of skills—skills that one will have to learn together with AI. The implications fall into three categories: predictions of the professional contributions that an AI-infused culture will value, the skills necessary for those contributions, and the ways AI will transform learning itself and its environments.
Until recently, most learning environments within schools of architecture still followed principles invented in Victorian times as a response to the beginning of industrialization. Not by coincidence, the typical amount of time involved in architectural education equates to Sennet’s ten thousand hours of apprenticeship by learning eight hours per day, five days per week, for five years. In many cases, a typical classroom still contains as many students as one teacher can instruct, and we still teach as though students are training for only one role that they will perform for their entire lifetime, for which they must memorize a body of knowledge that is a scarce resource.
Before digitalization, schools were places of exclusive access to knowledge. Schools were designed as a kind of Wunderkammer of a profession, with the goal of preparing students for any situation they might encounter in their professional lives. Therefore, schools had to provide a condensed simulation of reality on which students could be trained, tested, and certified. The standard curriculum in architecture still resonates with such motivations. In design studios, students repeatedly arrange, in increasing complexity, a set of spatial queries onto a virtual site reviewed by the studio’s professors. The standard studio outcome contributes only to a virtual, simulated context that is valued only as proof of mastering a set of skills of a prospective profession. Such a simulation of designing a building never reflected the reality of architectural practice. In reality, a team of architects designs a building in a far more extended period than a studio provides. However, the studio had to compress for students the wonders of their prospective buildings to come.
Digital technologies have enriched learning environments enormously, and AI systems might be the last melt below the tip of an iceberg. Classes based on a lecture format are a great example. Digital lectures, once recorded, can be replayed infinite times to infinite participants and provide their content asynchronously at any location. It is not necessary and no longer efficient to watch lectures gathered at one place at a dedicated time. Today’s Massive Open Online Courses (MOOCs) make knowledge accessible to remote locations or individual circumstances that might otherwise have precluded education plans, and are also available for marginal fees as a vastly greater number of participants share costs.
Available anywhere with an internet connection, a student can choose the best available content from the best teacher from a global collection. In response, Donald Clark, an expert on teaching methodologies, proposed the concept of “flipped lessons,” or courses where students watch lectures for homework and use classroom time to apply their content to practice. In this model, a teacher’s role flips from instructor to mentor or tutor in an interactive practice, serving as a curator to turn abundance into comprehensible lessons. In this scenario, teachers curate content that provides a platform for studying, and courses become stages for a particular discussion. As a result, students become constructive participants who shape innovation through projects analogous to the format of inquiry-based learning.
This is precisely where AI systems now step in. Already, in daily life, Google responds to queries with AI-generated summaries. The same AI linked to the interface of a chatbot turns into a personalized teaching assistant with an encyclopedic knowledge of the curriculum one needs to master. Trained with the data of one student’s life, the bot will understand—possibly better than the student themself—the most suitable approaches to their learning, such as the times of day when they are most receptive and when they are best left to relax for their emotional well-being. Mentors can use AI to translate a course’s content into personalized programs based on their own unique experiences and preferences. The bots can adapt to each student’s level of knowledge and speed of learning, sharpen their skills, and improve weak spots. Freed from a one-size-fits-all pace of knowledge transfer, and with AI that can augment missing skills, a class can be diverse, assembled from students with different skill levels based on their intent to contribute. In similar ways, grading can be much fairer, at once tailored to the individual student’s learned skills while also subtly leveraged against a globe-spanning database of comparable assignments.
Design environments require less of a chat bot than a drawing bot. With the encyclopedic knowledge of a building—including legislative codes, material features, structural behavior, building protocols, and so on—such a drawing bot would augment any professional knowledge onto the lines, shapes, or blocks we draw. Freed from the need to notate in conventional media and equipped with an XR interface, you can immediately draw or better choreograph architecture in space. Freed from the need to instruct by simulating a given knowledge, design studios can finally become places to design knowledge. Students can join a school not to learn but to study—in line with the classical notion of a university in which students do not learn today's facts but study future challenges to build their careers ahead. When knowledge is freely accessible anywhere at any time, the value of knowledge will increasingly lie in its authenticity and original contribution.
So, why should you still gather in a studio to design together? Because in the same way that one network can run with multiple instances, we can design now with many hands. Never before could designers jointly draw multiple responsive shapes simultaneously.
Unlike the modernist who needed to craft a single thing ten thousand times, and then again and again, designers in an AI-infused culture will design a single thing in ten thousand different ways. AI technologies offer us a new world of knowledge that is plural, non-linear, and non-repeatable by nature. The skills necessary to craft unique, authentic knowledge through the fusion of human and artificial intelligence coined a guideline for K-12 education six years ago as the “four Cs”: Communication, Collaboration, Critical Thinking, and Creativity. To immerse ourselves into the new design media, architects should similarly emphasize emotional intelligence, soft skills, and cognitive flexibility.
Wilson and Daugherty, scholars on the future of learning, elaborated on the fusion between humans and AIs: within augmented working environments, communication translates into the skill of an intelligent inquiry––knowing how best to ask questions to an AI agent. Collaboration becomes the capability of reciprocal learning, like teaching AI agents new skills and in turn learning AI-enhanced processes. Creativity depends first on the skill to design processes to increase available time for distinctively human tasks and learning. In augmented environments, critical thinking is exercised by integrating judgment, choosing a cause of action amid machine uncertainty, and endless rethinking—such as developing mental models of AI agents that improve collaboration outcomes or correct bias.
When asked what resources he needed for the 1990 founding of the Berlage Institute, one of the leading architecture schools of its time, Herman Hertzberger replied, surprised: “For a school, you don’t need anything. A school is a movement. It is a place of study, communication, interaction, support, and presence.” For most of history, schools have not been places to instruct or simulate an environment for problem-solving. They always have been places of confidence in the questions of the future.
When the work that the architect was known for has been automated, they will be freed from their craft and can focus on what they should be concerned about: the housing crisis, the climate crisis. Architecture schools will be freed from teaching drawing and other forms of notation, and studios can become places to envision and design proposals for the future. And we cannot have enough of such proposals; the challenges to the built environment are daunting. According to calculations by UN-Habitat, to address the housing crisis we need to build the equivalent of the existing US housing stock each year, across the globe. At the same time, according to the Intergovernmental Panel on Climate Change (IPCC) report, we need to find ways to reduce the carbon emissions of what should be a doubled building stock to 10 percent of today's emissions. Combined with the twenty-fold increase of building production and the cap to 10 percent of the current emissions, we need to teach future architects how to design and operate buildings some two hundred times more efficiently than we do today.
Soon, networks like DALL·E 2 will fully automate any aspects of notating a building, from a sketch to plans to the codes for robots to fabricate and build. Finally! When AIs can draw anything, architects will have the resources to design what we need more than anything. And more than anything, we need to design new architectures.
DANIEL KOEHLER >>
PLATFORM: TEACHING FOR NEXT >>
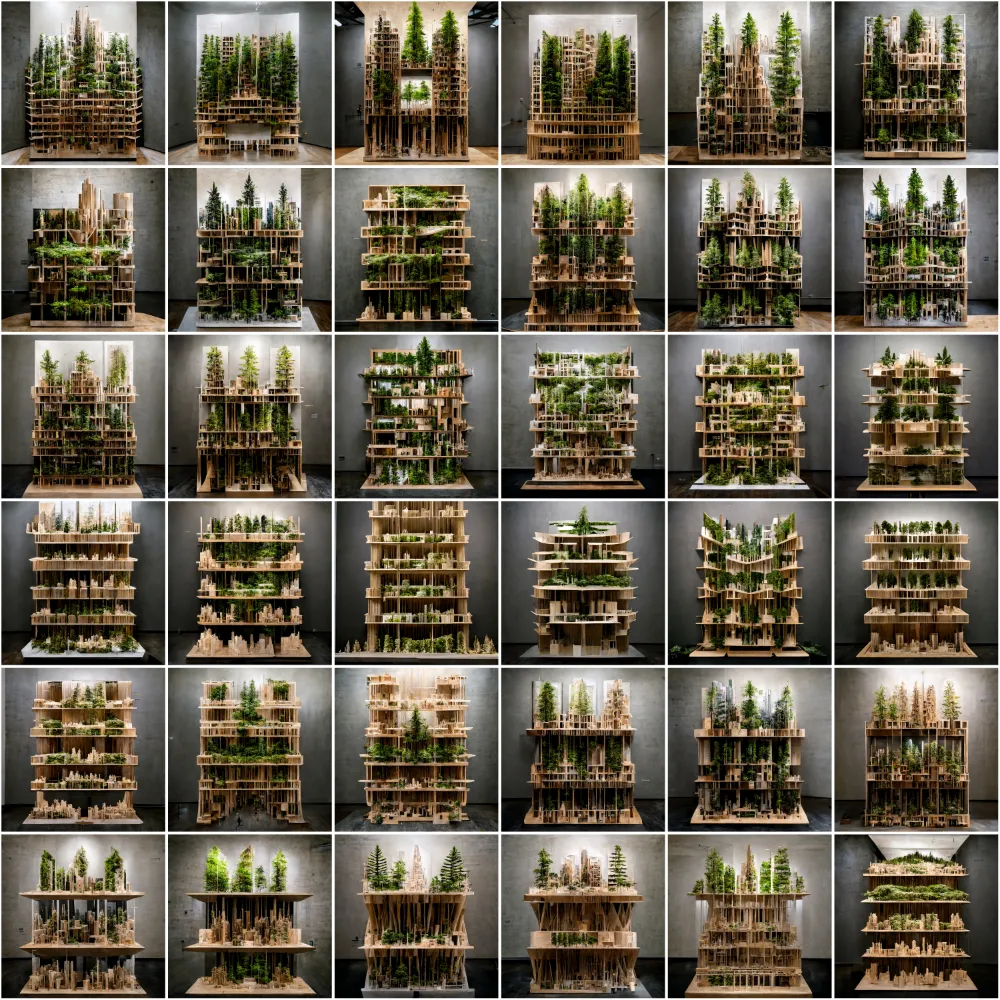
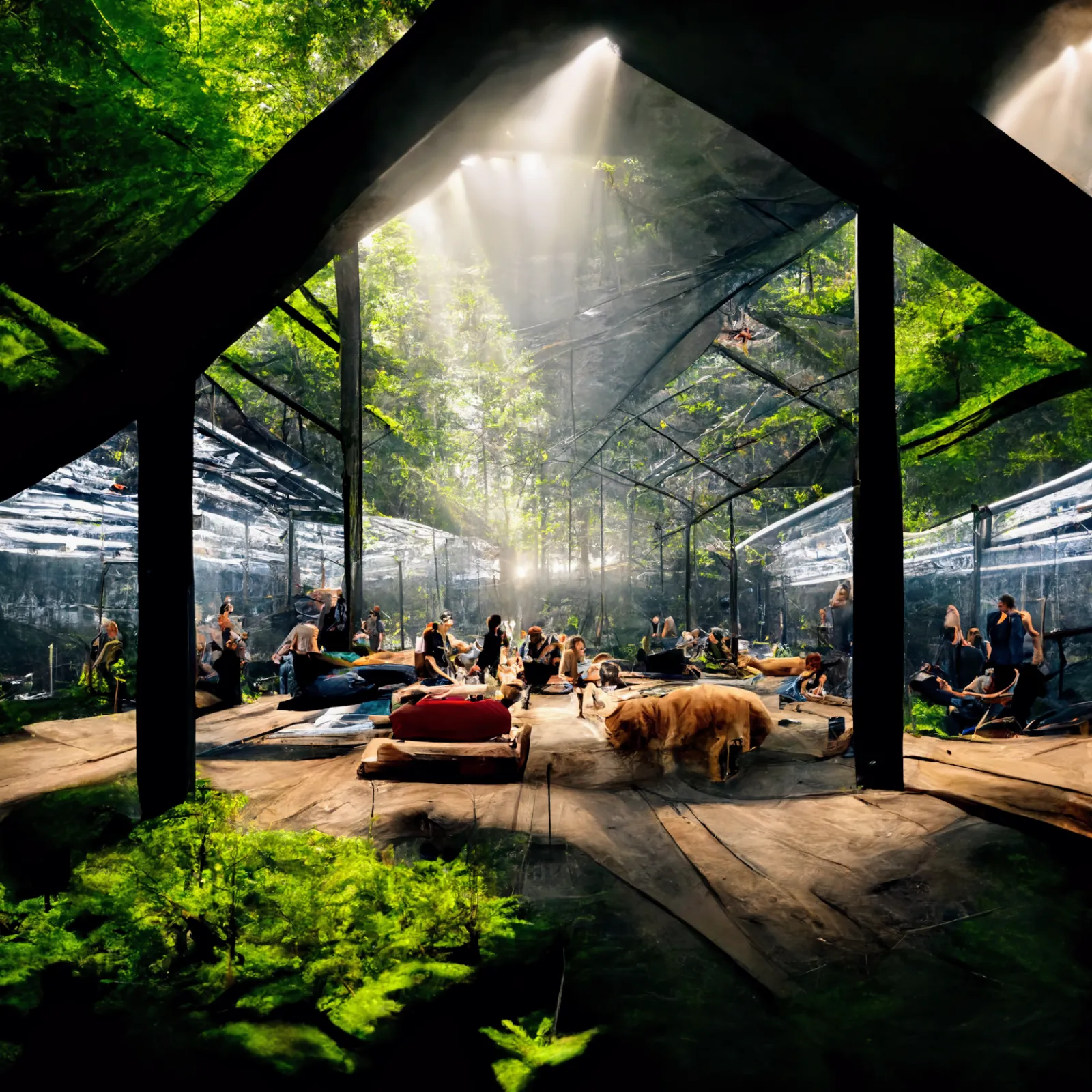
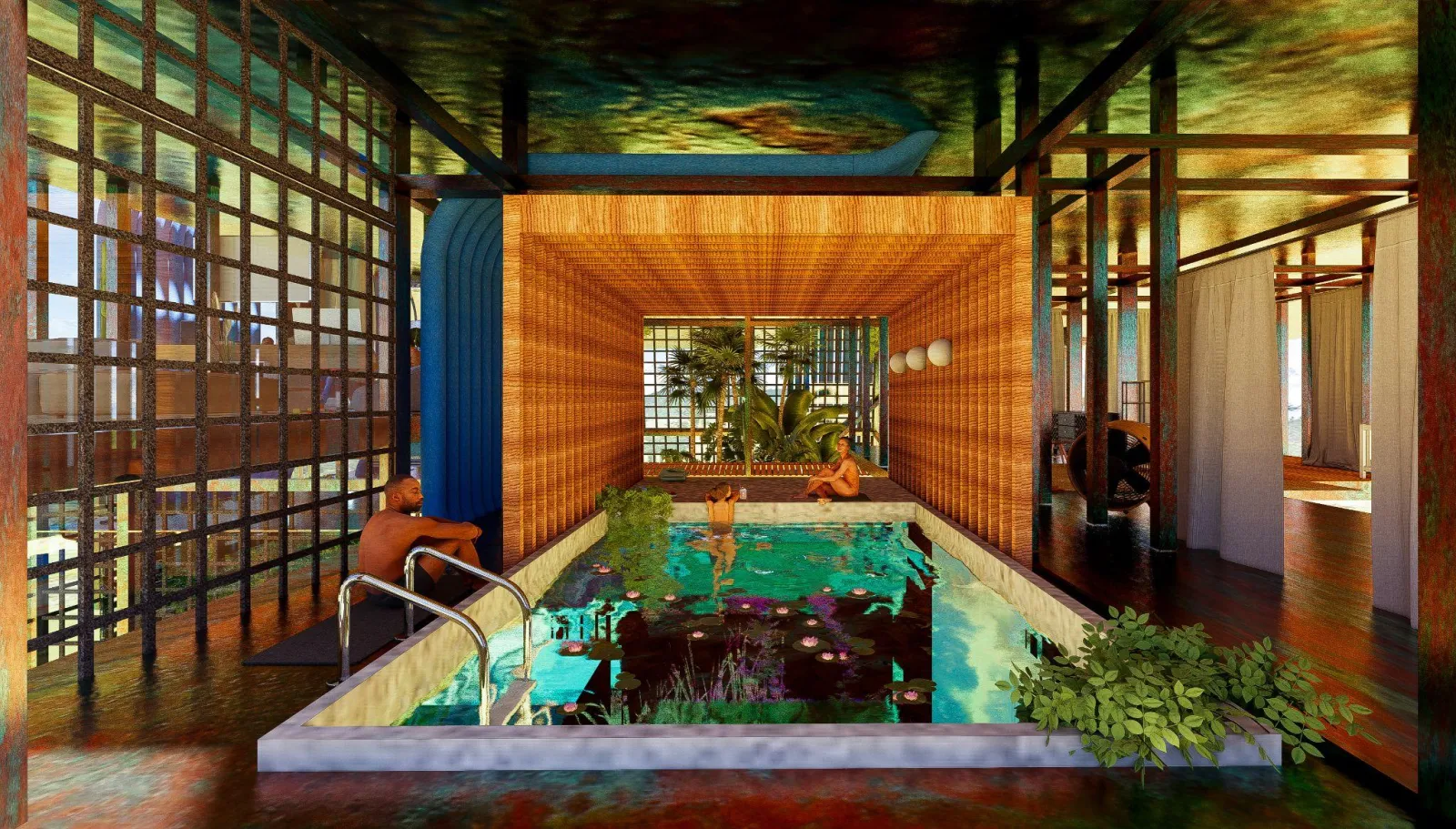
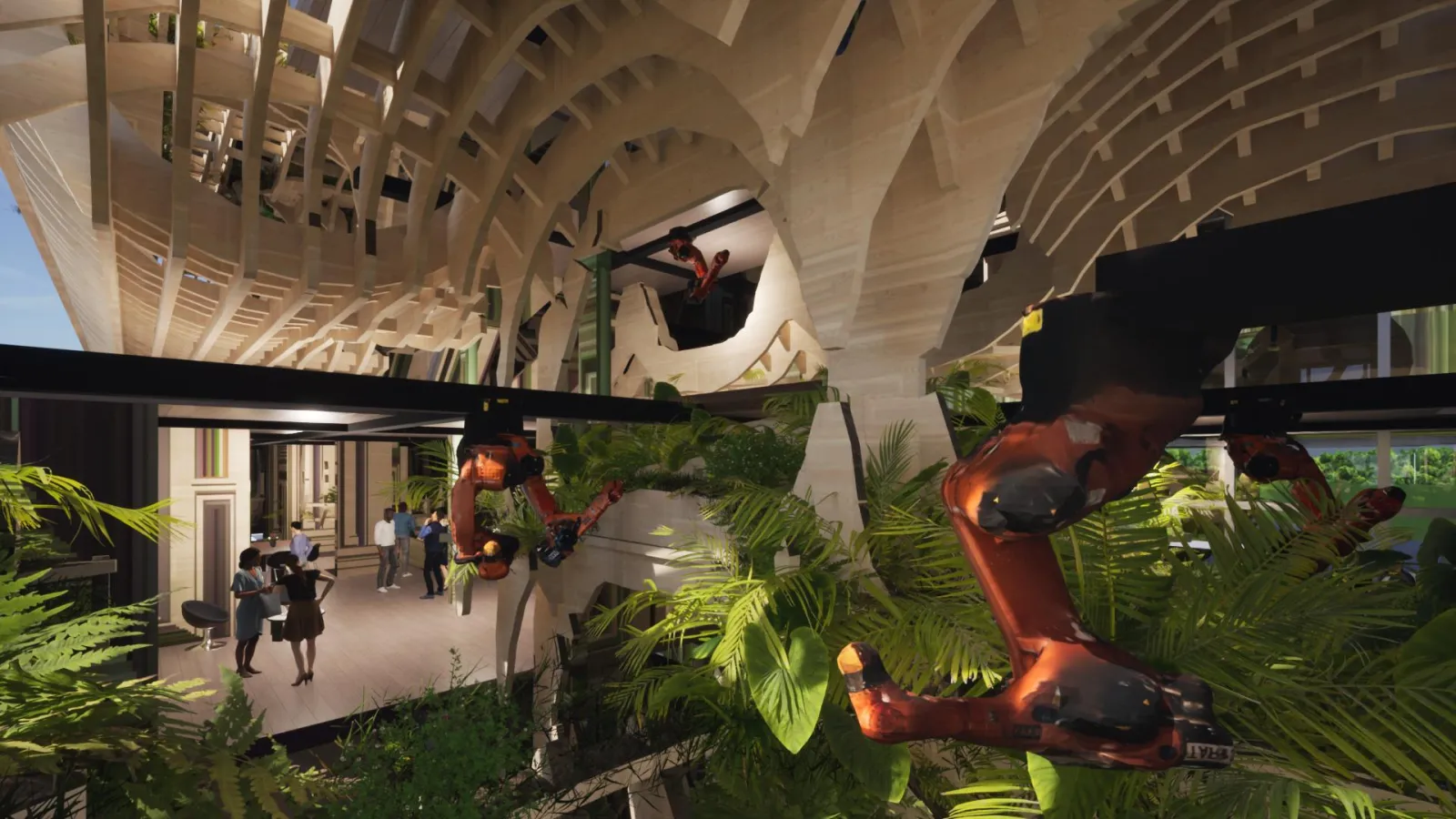